How Eco-Warrior Bots Use ML
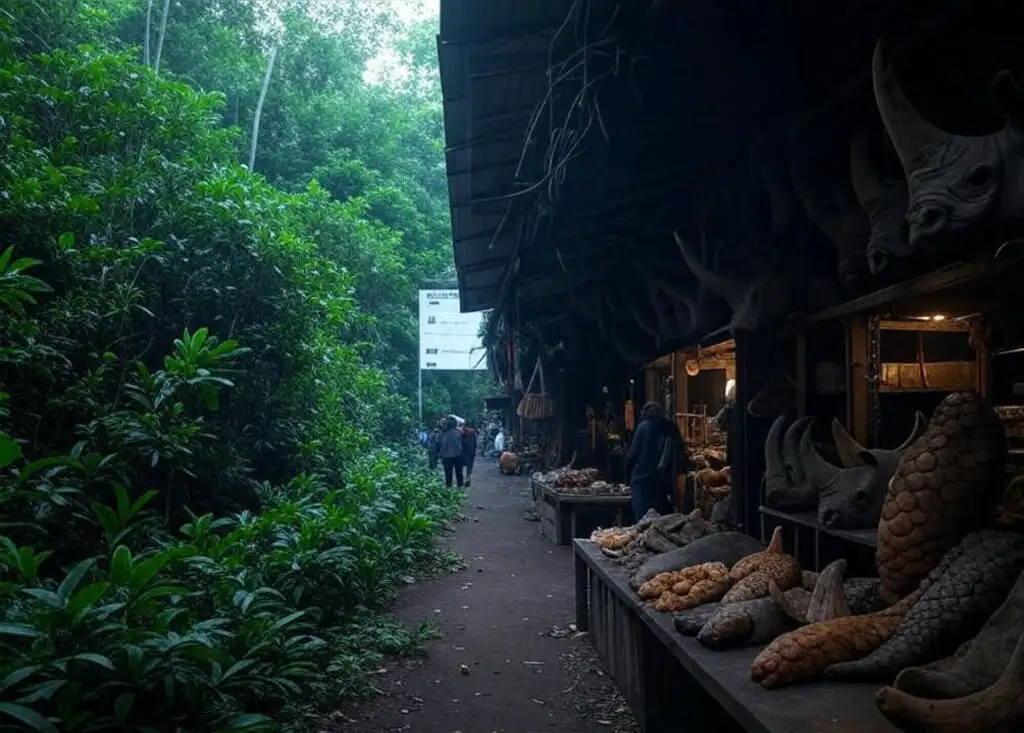
Eco-Warrior Bots are AI tools designed to combat illegal wildlife trafficking on social media by leveraging machine learning. They analyze large amounts of data to spot suspicious activities, helping conservationists and law enforcement act faster.
Table of Content
- How Eco-Warrior Bots Use ML
- Real-World Impact
- Challenges and Future Potential
- Detailed Analysis of Eco-Warrior Bots and ML in Tracking Illegal Wildlife Trafficking on Social Media
- Background and Context
- Machine Learning Techniques Employed
- Real-World Applications and Case Studies
- Challenges and Limitations
- Future Potential and Integration
- Conclusion
Content Identification
These bots use deep learning, specifically convolutional neural networks (CNNs), to identify images or videos of wildlife or their products, like rhino horns or pangolin scales, in social media posts. For example, they can distinguish between a pangolin in the wild and one in a market setting.
Text Analysis
Using natural language processing (NLP), the bots scan text in posts, captions, and comments to detect language linked to trafficking, even when traders use code words like “ox bone” for ivory. This helps uncover hidden illegal activities.
Data Mining and Filtering
The bots collect data from platforms like X, Facebook, and Instagram, filtering out irrelevant posts to focus on potential trafficking content. This process uses ML to narrow down the data for deeper analysis.
Pattern Recognition
By analyzing combinations of images, text, and user metadata, ML helps identify networks or recurring behaviors, such as groups of users frequently posting about exotic pets, which could indicate trafficking.
Real-World Impact
Studies show ML is already making a difference. For instance, a study detected over 90% of pangolin trade instances in images from online platforms using deep learning (Detecting wildlife trafficking in images). Another found 53 suspicious X posts about ivory sales in 14 days using ML and keyword filtering (Use of Machine Learning to Detect Wildlife Product Promotion).
Challenges and Future Potential
Challenges include data gaps for rare species and traffickers evading detection by using encrypted apps. Privacy concerns also arise, requiring collaboration with social media companies. However, the future looks promising, with bots potentially providing real-time alerts and integrating with systems like the Coalition to End Wildlife Trafficking Online, which has removed millions of illicit posts.
Detailed Analysis of Eco-Warrior Bots and ML in Tracking Illegal Wildlife Trafficking on Social Media
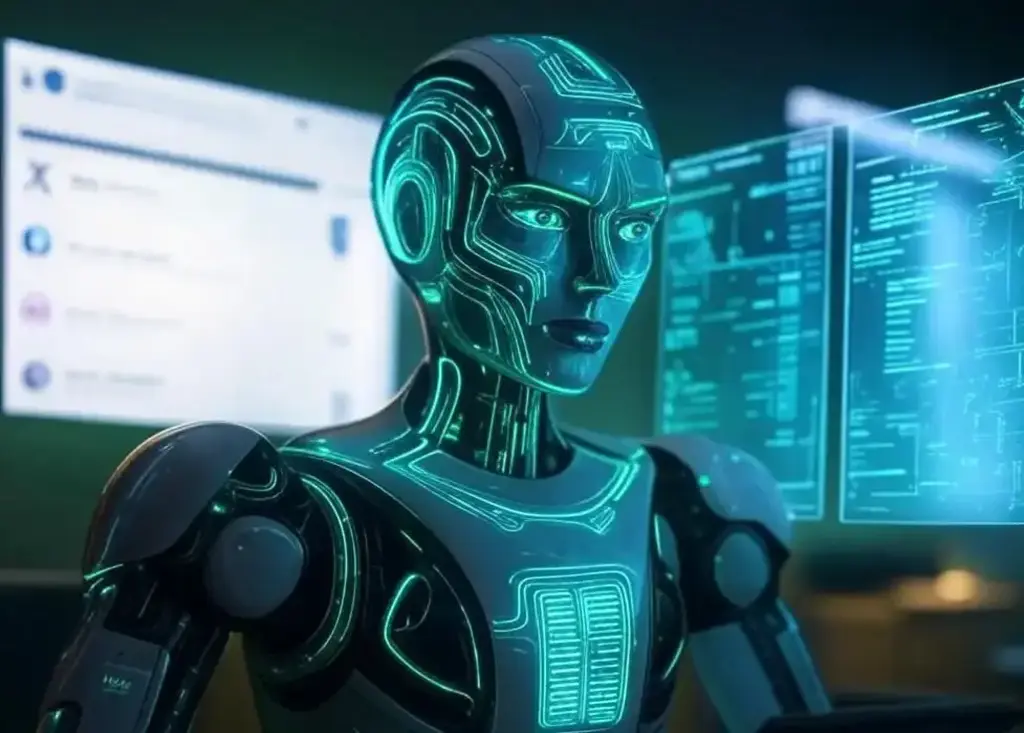
Eco-Warrior Bots represent an innovative application of machine learning (ML) to address the growing challenge of illegal wildlife trafficking on social media platforms. In 2025, this technology is emerging as a critical tool in conservation efforts, leveraging advanced AI to monitor and mitigate the online trade of endangered species and their products. This section provides a comprehensive overview, including technical details, real-world applications, challenges, and future potential, supported by research and examples.
Background and Context
Illegal wildlife trafficking has increasingly shifted to online platforms, exploiting the accessibility and vast reach of social media. This shift poses significant challenges for traditional manual monitoring methods, which struggle to handle the scale and complexity of data.
Eco-Warrior Bots, conceptualized as AI-powered tools, use ML to automate detection and analysis, offering a scalable solution to this global conservation crisis. The focus is on platforms like X, Facebook, and Instagram, where traffickers post images, videos, and text to advertise and sell wildlife products.
Machine Learning Techniques Employed
The core of Eco-Warrior Bots lies in their use of ML, particularly deep learning and natural language processing (NLP), to process unstructured data. The following table outlines the key techniques and their applications:
Technique | Description | Specific Application/Example |
Deep Learning (CNNs) | Uses neural networks to identify visual content in images and videos. | Trained to recognize pangolins in market settings, achieving over 90% accuracy in trade detection. |
Natural Language Processing | Analyzes text to detect code words or phrases linked to trafficking. | Identifies terms like “ox bone” for ivory in X posts, flagging suspicious content. |
Data Mining and Filtering | Collects and filters social media data to focus on relevant posts. | Scrapes X, Facebook, and Instagram, using ML to discard non-relevant posts. |
Pattern Recognition | Analyzes user networks and behaviors to uncover trafficking patterns. | Identifies clusters of users posting about exotic pets, suggesting organized trafficking. |
These techniques operate within a three-stage framework proposed in research: mining (data collection), filtering (irrelevant content removal), and identifying (confirming trafficking-related posts). For instance, a study published in Conservation Biology (A framework for investigating illegal wildlife trade) detailed this approach, emphasizing automated monitoring.
Real-World Applications and Case Studies
Several studies illustrate the practical application of ML in this domain. A notable example is the use of deep learning to detect pangolins in images, as detailed in a paper from Biological Conservation (Detecting wildlife trafficking in images).
Researchers trained convolutional neural networks with images from iNaturalist, Flickr, and Google, focusing on traded and non-traded pangolin settings. The model achieved over 90% accuracy in identifying potential trade instances, particularly in marketplaces like wet markets or cages, though it struggled with dark or shaded images.
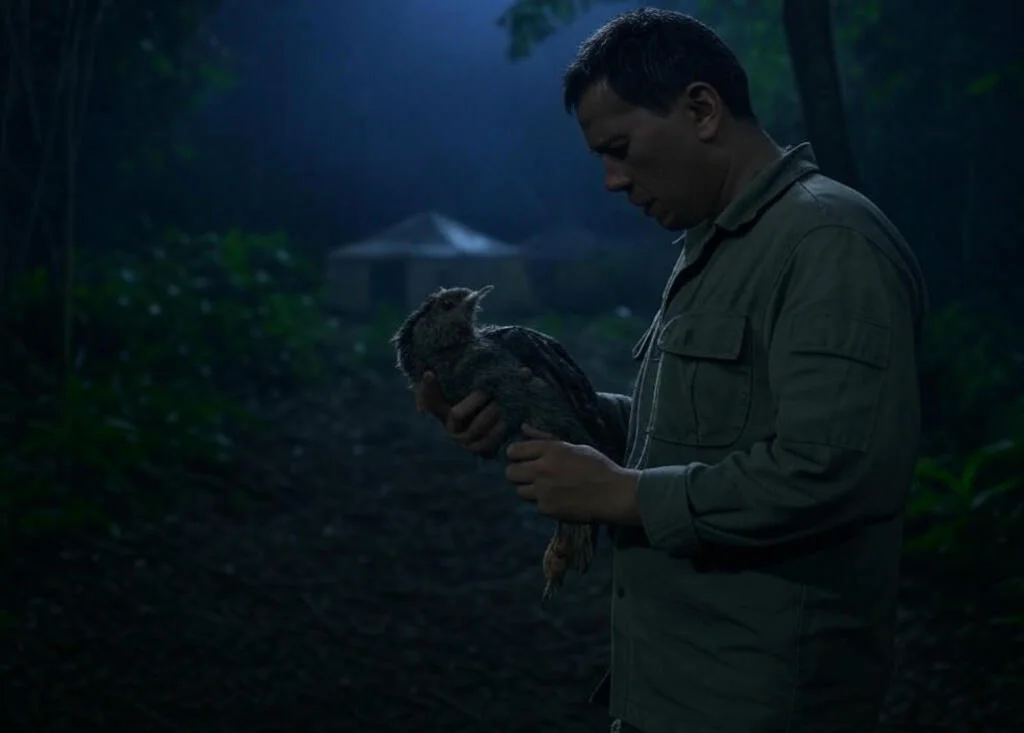
Another case involved Twitter (now X) data, where a study in Frontiers in Big Data (Use of Machine Learning to Detect Wildlife Product Promotion) used an unsupervised ML topic model combined with keyword filtering to detect suspicious posts. Over 14 days, they collected 138,357 X posts, identifying 53 suspicious posts from 38 unique users promoting ivory sales, though no pangolin-related promoted posts were detected, possibly due to rarity or method limitations.
These examples highlight the versatility of ML, from image analysis to text-based detection, and its integration with existing systems like the Coalition to End Wildlife Trafficking Online, which has removed millions of illicit posts through collaboration with tech companies.
Challenges and Limitations
Despite their potential, Eco-Warrior Bots face several hurdles. Data gaps are a significant issue, particularly for rare species or obscure products, as insufficient training data can reduce model accuracy. For instance, the pangolin detection study noted challenges with images having dark colors and shaded areas, suggesting a need for more diverse datasets.
Traffickers also adapt by using encrypted messaging apps like WhatsApp or shifting to less-monitored platforms, requiring continuous evolution of the bots.
Ethical concerns are another critical area, especially regarding user privacy. Automated monitoring must comply with regulations like the EU General Data Protection Regulation, necessitating partnerships with social media companies to ensure ethical data use. Research suggests these challenges can be addressed through citizen science initiatives, like Zooniverse (Zooniverse), where users label images and text to expand training datasets.
Future Potential and Integration
Looking ahead, Eco-Warrior Bots could revolutionize conservation by providing real-time alerts to law enforcement, conservation groups, and platform moderators. Enhanced with web search capabilities and X post analysis, they can cross-reference findings with external sources or track sentiment trends, such as spikes in posts about a specific species signaling increased trafficking activity. This could prompt targeted interventions, aligning with initiatives like CITES (Decisions 17.92 and 17.93), which advocate for partnerships with AI scientists and social media platforms.
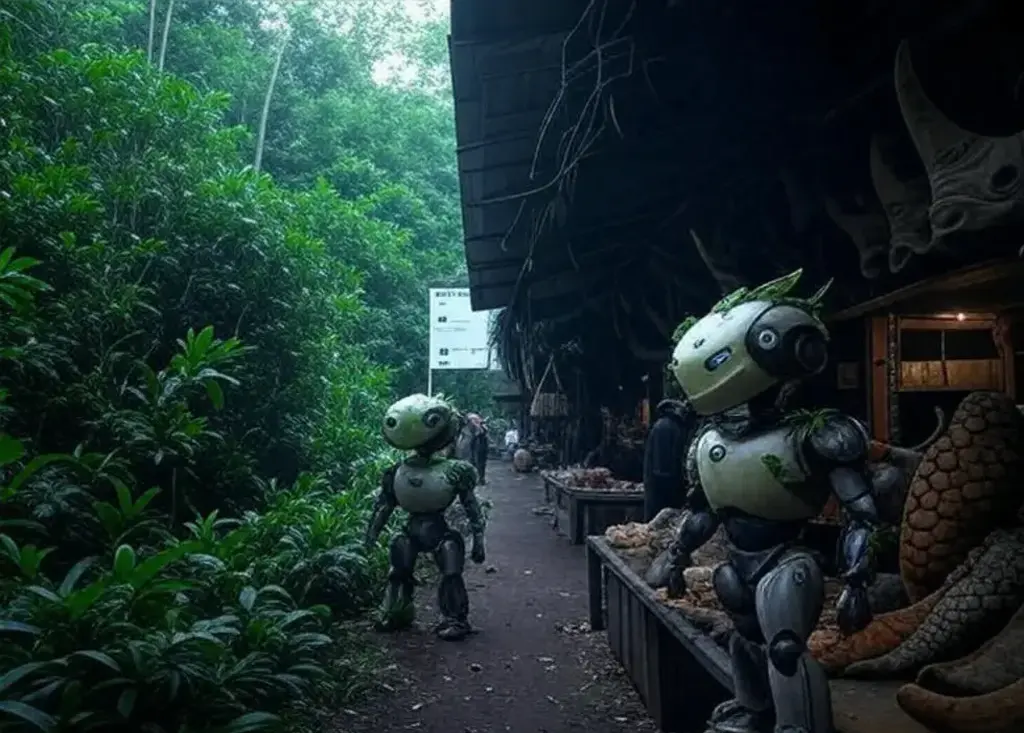
The integration with existing systems, such as the Coalition to End Wildlife Trafficking Online, suggests a collaborative future where bots not only detect but also facilitate the removal of illicit content at scale. Continuous development, supported by advancements in ML, could make these bots a cornerstone in protecting biodiversity, particularly for heavily trafficked species like pangolins, rhinos, and elephants.
Conclusion
Eco-Warrior Bots, powered by ML, offer a promising approach to tackling illegal wildlife trafficking on social media. These bots address a critical conservation challenge by leveraging deep learning for image analysis, NLP for text interpretation, and pattern recognition for network detection.
While challenges like data gaps and privacy concerns persist, ongoing research and real-world applications demonstrate their potential, such as pangolin detection and ivory sale flagging. In 2025, this technology is still emerging, but its evolution could significantly enhance global efforts to protect endangered species.
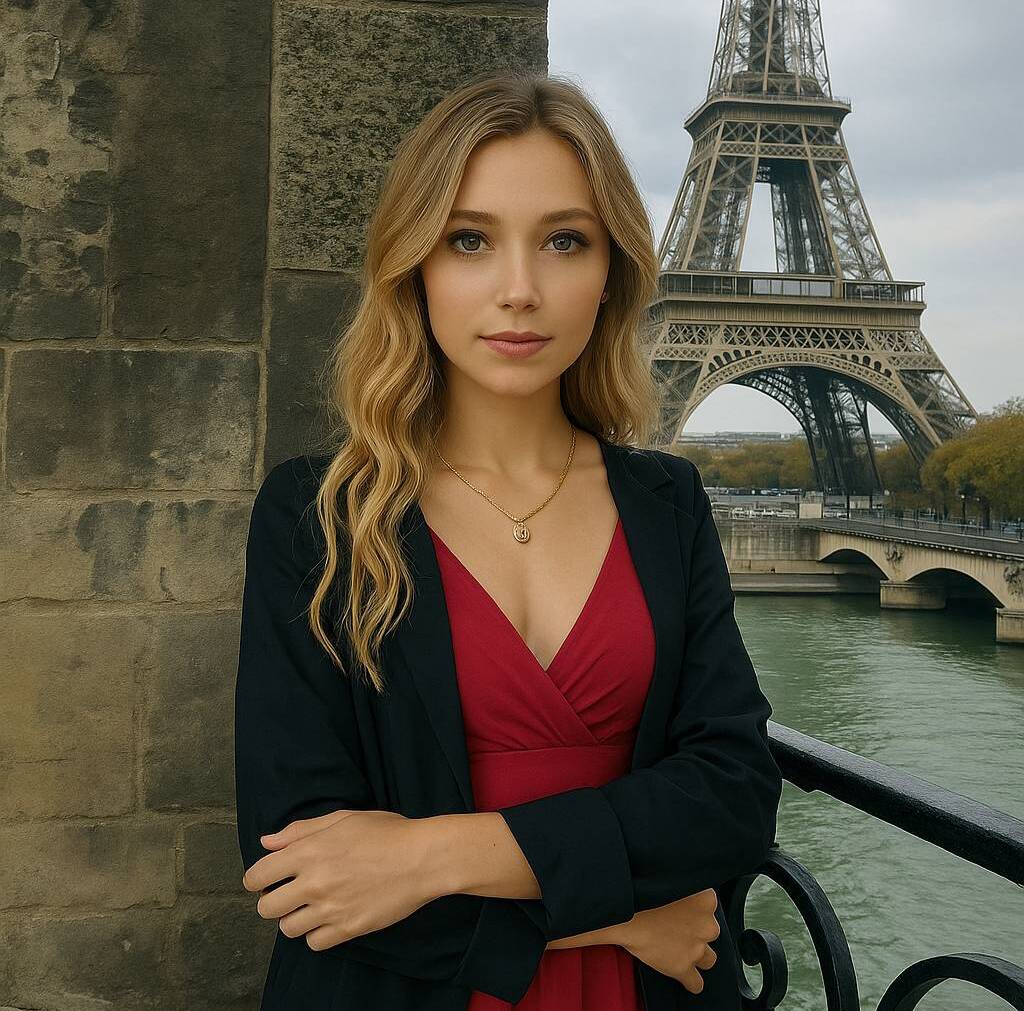
Passionate AI enthusiast and writer, I explore the latest advancements, trends, and ethical implications of artificial intelligence. Through my blog, I aim to simplify complex AI concepts and spark meaningful conversations about its impact on our future.